Document Type : Original Article
Authors
1
Department of Industrial Engineering, Faculty of Engineering, Kharazmi University, Tehran, Iran
2
School of Industrial Engineering, College of Engineering, University of Tehran, Tehran, Iran
Abstract
The economic production quantity (EPQ) model considers the production rate, demand rate, setup costs, holding costs, and shortage costs to find the production quantity that minimizes the sum of these costs. The goal is to balance the costs associated with production, holding inventory, and potential shortages. In this paper, two objectives include the costs of production and ordering and others in a separate objective function. In the objectives of the other costs, The cost of storage space as a supply is defined to be minimized. This study considers scrap and reworks in the EPQ model. This inventory model accounts for many items on a single machine. The production capacity is reduced, and there are shortages when only one machine exists. By determining the quantities of the products produced by the manufacturing facility, the storage space for each product, cycle time, and product scarcity, we can reduce both the overall cost and the supply cost of warehouse space due to non-linearity and the inability to solve commercial software in large dimensions, a multi-objective meta-heuristic algorithm, namely the non-dominated sorting genetic algorithm (NSGA-II), is used. The findings are further validated using the non-dominated ranking genetic algorithm (NRGA). Also, the obtained Pareto front is studied with several indicators. To perform these two algorithms at the best condition, we employed the Taguchi approach and related orthogonal arrays and performed algorithms for each array considering several factors. Also, to validate the mathematical model, we used the augmented epsilon-constraint method executed in the GAMS environment. It is clear that GAMS commercial software yields better results; however, these two algorithms are justifiable when the problem becomes bigger. Finally, by performing a sensitivity analysis for these indicators and the objective functions, the behavior of the proposed algorithms is compared and examined in detail. Also, the superior algorithm is chosen using the TOPSIS as a multi-criteria decision-making method. Numerical examples show how the presented model and the proposed algorithms may be used efficiently. A surveying literature review clarifies that the related objective functions, constraints, and solution approaches have not been investigated until now.
Graphical Abstract
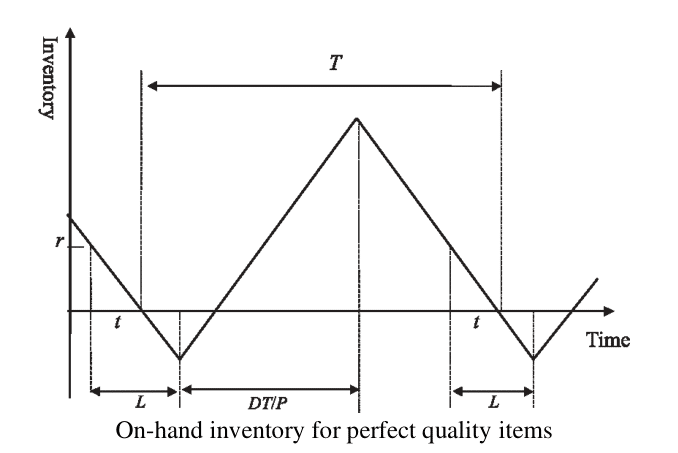
Keywords
Main Subjects